Integrative data analysis (IDA) refers to a set of strategies in which two or more independent data sets are pooled or combined into one and then statistically analyzed. IDA approaches differ from and offer advantages over other methodological techniques that also strive to build cumulative knowledge bases, such as meta-analysis.
In meta-analysis, summary statistics across multiple studies are pooled together. Because IDA techniques pool original raw data, there is no loss of individual information as found within meta-analytic approaches, which allows researchers to find out what works, for whom, and in which contexts. In addition, the use of IDA affords expanded inquiry within many areas of health behavior research. IDA can be used to incorporate big data that were not originally intended for the examination of theoretically relevant measures. For example, searches on Google for health-related topics could be used as an objective measure of information seeking that could supplement what is gleaned from a self-report data source such as the Health Information National Trends Survey (HINTS).
Data integration typically takes one of two forms:
- merging data by common data elements (units of information that are shared or widely used across data collection efforts.), where these elements are often multi-item scales or indices but can be individual items; or
- linking data sets through a common factor at the record level (e.g., linking across data through demographic information) such as that seen in the Surveillance, Epidemiology, and End Results (SEER)-Medicare data set, or at multiple levels such as the environmental level (e.g., linking state- or county-level information with individual-level data).
The Behavioral Research Program seeks to promote the use of IDA to answer novel cancer control questions to accelerate scientific discovery.
Funding Opportunity Announcements
Title | Announcement # | Expiration Date | Contact |
---|---|---|---|
NCI Small Grants Program for Cancer Research | PAR-20-052 (NCI Omnibus R03 Clinical Trial Optional) | January 08, 2023 | Crystal Wolfrey Email: wolfreyc@mail.nih.gov |
View Past Funding Announcements
Publications
- Special issue of Psychological Methods, published June 2009
Contact
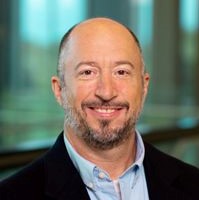